Target Detection in Hyperspectral Remote Sensing Images using Machine Learning Techniques - دانشکده فنی و مهندسی
Target Detection in Hyperspectral Remote Sensing Images using Machine Learning Techniques
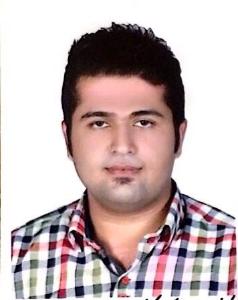
نوع: Type: thesis
مقطع: Segment: masters
عنوان: Title: Target Detection in Hyperspectral Remote Sensing Images using Machine Learning Techniques
ارائه دهنده: Provider: Arash Ghiyas Yazdi
اساتید راهنما: Supervisors: Dr. Hassan Khotanlou - Dr. Yousef Rezaei
اساتید مشاور: Advisory Professors:
اساتید ممتحن یا داور: Examining professors or referees: Dr. Muharram MansooriZadeh - Dr. Morteza Heydari Mozaffar
زمان و تاریخ ارائه: Time and date of presentation: چهارشنبه 30 بهمن ماه 1398 ساعت 12
مکان ارائه: Place of presentation: آمفی تئاتر دانشکده مهندسی
چکیده: Abstract: Satellite imagery is known as remote sensing imagery. Target detection is one of the most important topics in a variety of fields, including military, agricultural, and geological. Detecting a target, such as a plane or finding landmines in war zones, finding groundwater resources are all useful in identifying targets. Because every material has a unique spectrum for identification in hyperspectral images. Timely and accurate detection of a target is very important, and since humans may be mistaken in identifying some of the targets, while an intelligent system that can effectively detect objects. Recently modeling in the field of artificial intelligence in hyperspectral image processing can include models based on deep neural networks and convolutional neural networks. In this study, a method based on deep convolutional neural network for problem detection, classification and target identification in hyperspectral remote sensing images is presented, which generally consists of two parts: pre-processing and detection. The preprocessing part consists of a network for pre-training the data, and the detection part consists of the first part using the pixel-pair model to obtain the difference of each pixel with its neighbors and the second part comprising two classifier based on a fully connected layer in deep canonical neural networks and it is a support vector machine that performs well in each category and performs the detection and classification of images with great accuracy. The pixel-pair model is that if the central pixels are adjacent to the same pixel as a member of a class, they are in the same class and if they are not members of a class, they will be different class members. The network selected for pre-training data is the Restricted Boltzmann network. The existence of this network as pre-training makes the difference in feature extraction and learning. In this study, hyper-spectral images are classified into two classes of "target and non-target" according to the image classes. However, given the application of the Restricted Boltzmann network in relation to dimensionality reduction, a comparison between the performance of the network with the personal component analysis method is accepted. Finally, the proposed method is applied to Salinas and Indian Pines datasets. At last, proposed method was applied and evaluated by some metrics like Area Under The Curve to describe the performance
فایل: ّFile: Download فایل